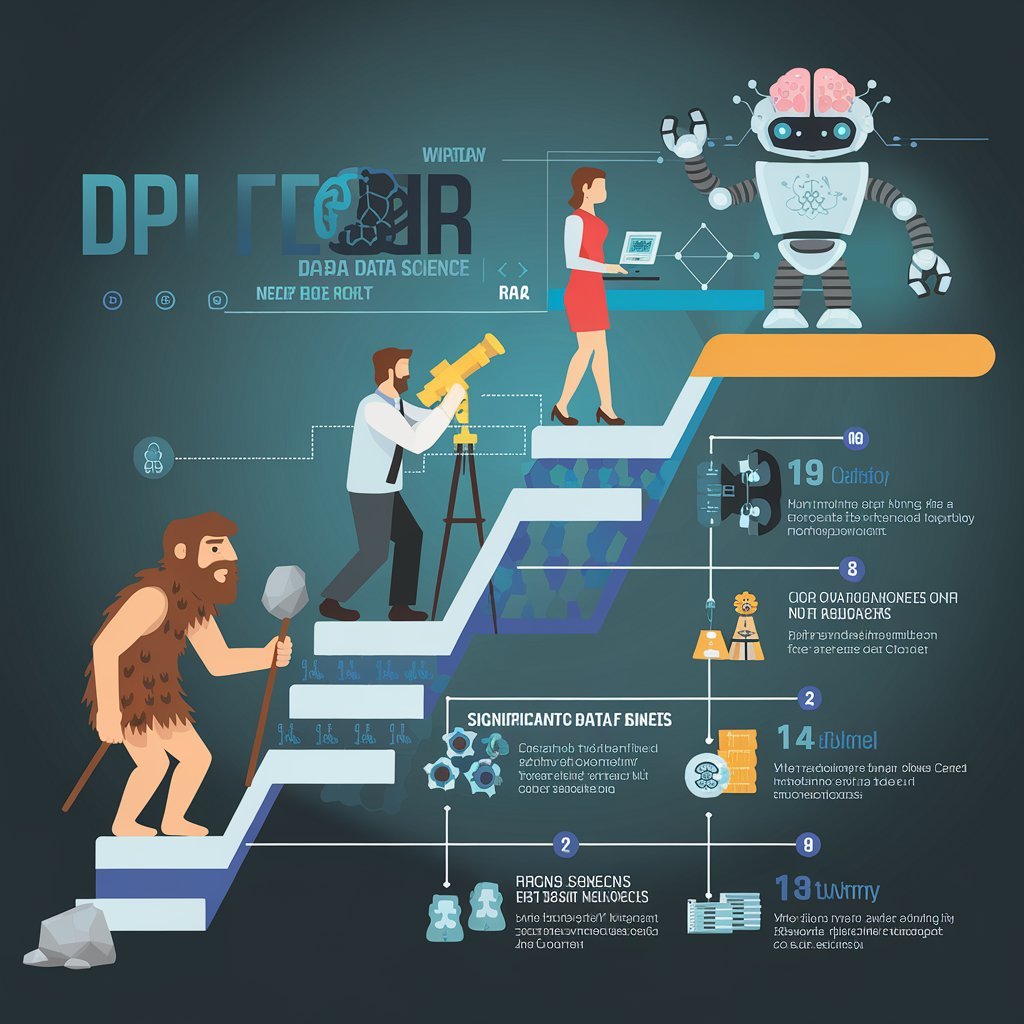
Data science has emerged as one of the most important and dynamic sectors during this age of the digital world. From its roots through statistics and computer science to its very current presence in virtually every sector, there is no sense in which the growth of data science parallels its peer fields. Advanced analytics are driving the advancement and proper decision-making, optimization of operations and, most importantly, the transformation of industries with the vital use of data science. We will consider the beginning of Data science and discuss its key development stages followed by why data science is important in today’s world of data.
Origin of Data Science
The root of data science is in statistics and mathematics. Indeed, for many decades, mathematics served as a model for understanding data and predicting their behavior. In the mid-20th century, the advent of computers empowered computational statistics, hence providing easier processing of large datasets.
With an increase in data collection, the term “data science” started coming into usage in the 1960s and 1970s but was not identified as a distinct field of study. It is only in the early 2000s, with the shaping of data science by merging computer science, mathematics, statistics, and domain-specific knowledge, that the term has started to gain shape. The symbiosis of the above discipline enabled business and organizations to recognize new potential for analyzing and leveraging the massively increasing volume of data.
Evolution of Data Science: Key Milestones
1. Big Data Boom
The real thrust of modern data science was actually heralded by the explosion of digital data that had come about in the first two decades of the 21st century. From the proliferation of the internet and of mobile devices as well as from social media and cloud computing, huge amounts of unstructured data were being generated, amounts unprecedented in previous periods. This “big data” was to form the bedrock upon which the rise of data science was founded, extracting meaningful insights from the ever-mounting tide of data.
Companies like Google, Amazon, and Facebook made it very easy to use big data to make their companies more competitive. Google’s early application of data science is the PageRank algorithm that ranks web pages according to relevance. During this time, Amazon was one of the first companies to build individualized product recommendations with user behavior and transaction data.
2.Advancements in Machine Learning:
Machine learning has been one of the biggest drivers of innovation in data science. The concept of machine learning can trace back to the 1950s, but breakthroughs in computing power and large datasets at the close of the 2000s are what have finally made algorithms in this field show real major breakthroughs.
Machine learning is the way in which computers are making sense of the patterns on the data they load without explicit programming. Its relevant application can be noted in cases such as speech recognition, image categorization, fraud detection, and predictive analytics. Netflix uses this to predict user preferences, while financial institutions depend on it to detect fraudulent transactions.
3. Deep Learning and AI
Deep learning represents a subset of machine learning and has done nothing but revolutionize the world of data science. Deep learning models, centered on artificial neural networks, have succeeded in NLP, image recognition, and autonomous vehicles to a remarkably impressive extent. Deep learning algorithms process vast amounts of data by simulating the brain’s neural networks.
Deep learning became a breakthrough towards the end of the last decade, especially after the growth of big data and increased computational power. This brings innovation in sectors like health care whereby AI-powered systems that use medical images recognize diseases and propose treatment alternatives. A perfect case in point would be Google’s AlphaGo, which beats a Go world champion .
4. Cloud Computing and Scalable Data Processing
The development of data science is further bolstered by the rise of cloud computing as well.Through cloud platforms like Amazon Web Services (AWS), Microsoft Azure, and Google Cloud, businesses will be able to store, process, and analyze large datasets in scalable and cost-effective ways. These are the infrastructure bases that will enable the complexity of modern data science workflows, which often involve real-time data streams and large-scale machine learning models.
With cloud-based solutions, anyone can now simply access data science, no matter what size an organization is, as it could easily and affordably adopt complex tools and algorithms without significant investments in hardware.
Data Science and the World of Today
1. Data-Driven Decision Making
Business can store, process, and analyze large datasets in scalable, cost-effective ways through the help of cloud platforms such as AWS, Microsoft Azure, and Google Cloud. Such platforms can provide an infrastructure that enables the complexity of modern data science workflows-which normally involve real-time streams of data and large-scale machine learning models.
For example, financial organizations employ data science when planning to predict market trends, manage risks, and optimize the investment portfolio. In retail, the same insights could mean better knowledge about the behavior of customers, efficiency in the supply chain, and targeted marketing.
2. Improved Efficiency and Automation
In the manufacturing sector, or in any other sector, for that matter, leverages data science and machine learning models to automate repetitive tasks that also enhance the efficiency of operations.It enables the prevention of certain failures of the machinery by having data science-based predictive maintenance systems, which could ultimately save costs and avoid untimely downtime.
With data science, the logistics industry can perform route optimization by delivering goods. In a nutshell, with data science automation, transformation of industries will take place so that strategic activities can be set time off business operations while burdening the people less.
3. Customer Experience Improvement
Business starts using data science to come up with the most personalized and engaging customer experience possible. Companies can adjust their offerings and recommend their products by making use of personalization based on customer data. For example, services like Netflix and Spotify are making recommendations about content that a customer should watch or listen to based on user preference and make use of data science.
Data science in the e-commerce industry helps predict customer needs and provide users with customized product recommendations, thus increasing satisfaction with customers and retaining and garnering loyalty.
4. Innovation
Data science is a catalyst for innovation in many fields. In healthcare, it provides data-driven insights to give customized treatment plans and improve drug discovery as well as predict patient outcomes. Transportation is another area that’s becoming possible due to the application of data science and AI technologies such as autonomous vehicles, smart cities, and more.
Data science is solving some of the vital global concerns, including climate change. Predictive models help in understanding weather patterns, tracking deforestation, and optimizing sources of renewable energies. The promise of data science in enabling positive social change is pretty enormous.
Conclusion
It is actually the evolution of data science from its root being statistics to being a transformative technology as well as the importance of data science in a world dominated by data today. Organizations that continue to generate and collect an ocean of data will need data scientists and analysts, and the ability to interpret and analyze data effectively will no longer be a luxury but a necessity for businesses looking for an edge to survive the market.
Data science helps with enabling data-driven decision-making, improving the efficiency of operations, and enriching customer experience and is transforming the future shape of industry worldwide. As technology in the fields of machine learning, AI, and cloud computing advances, the profound effects data science could leave on the world are going to be told at various levels. The future of data science is very bright and cannot be overemphasized by enough words.